|
ACCESS THE FULL ARTICLE
No SPIE Account? Create one
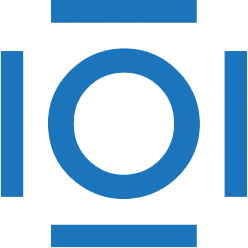
CITATIONS
Cited by 15 scholarly publications.
Data modeling
Eye
Neural networks
Optical coherence tomography
Optical discs
Image segmentation
Performance modeling