|
ACCESS THE FULL ARTICLE
No SPIE Account? Create one
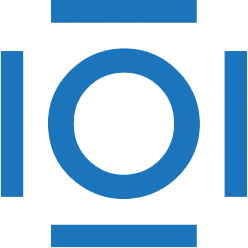
CITATIONS
Cited by 2 scholarly publications.
Image classification
Data modeling
Image analysis
Medical imaging
Image segmentation
Machine vision
Cancer