|
ACCESS THE FULL ARTICLE
No SPIE Account? Create one
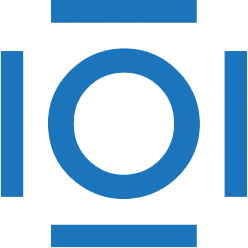
CITATIONS
Cited by 1 scholarly publication.
Chromophores
Principal component analysis
Near infrared spectroscopy
Absorption
Signal attenuation
Canonical correlation analysis
In vivo imaging