|
ACCESS THE FULL ARTICLE
No SPIE Account? Create one
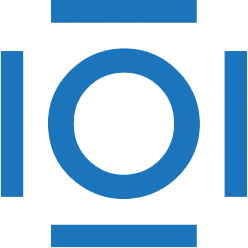
CITATIONS
Cited by 7 scholarly publications.
Skin
Melanoma
Blood
Collagen
Hyperspectral imaging
Machine learning
Absorption