|
ACCESS THE FULL ARTICLE
No SPIE Account? Create one
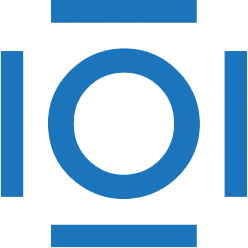
CITATIONS
Cited by 14 scholarly publications and 1 patent.
Tissues
Data modeling
Multispectral imaging
Blood
Injuries
Skin
Detection and tracking algorithms