|
ACCESS THE FULL ARTICLE
No SPIE Account? Create one
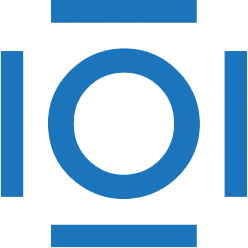
CITATIONS
Cited by 1 scholarly publication.
Signal to noise ratio
Atrial fibrillation
Hyperspectral imaging
Clouds
Data modeling
Remote sensing
Image analysis