1.IntroductionThere is a growing number of applications at both a clinical and consumer level where near infrared spectroscopy (NIRS) is used to noninvasively monitor tissue hemodynamics in skeletal muscle or cerebral cortex. By exploiting the capability of near infrared light to penetrate deeply into biological tissues and the specific spectral features of oxygenated and deoxygenated hemoglobin, it is in fact possible, from light attenuation measurements, to noninvasively estimate the molar concentration of these chromophores in the tissue under investigation, and then retrieve tissue oxygen saturation (). This parameter can be used as a biomarker for physio-pathological conditions in the clinics1 or as an indicator of skeletal muscle fatigue during exercise and sports activities.2 Due to the diffusive nature of biological tissues, estimate requires either discrimination of absorption and scattering contribution, as provided by the time domain (TD) NIRS or frequency domain (FD) NIRS,3 or the adoption of specific measurement strategy, like in the space-resolved reflectance (SRS) approach.4 Nowadays, the SRS approach is being used in many continuous wave (CW)- NIRS devices for clinical research and for consumer applications targeting skeletal muscle or brain hemodynamics. A recent database of available instruments can be found in Ref. 5. The characteristic feature of CW SRS NIRS is the possibility to noninvasively provide an estimate for —the so-called tissue oxygenation index or tissue saturation index—based on the spatial gradient of light attenuation detected at two wavelengths by two or three narrowly spaced detectors. The practical implementation of CW SRS NIRS brings to a simpler and cheaper device than FD NIRS or TD NIRS that can naturally provide . However, the CW SRS NIRS approach has some basic limitations mainly related to the lack of a direct estimate of the reduced scattering coefficient , to the heterogeneity of the medium, and to the use of simplifying assumption on modeling boundary conditions for reemitted photons. A first fundamental assumption of the SRS method is in fact the a priori knowledge of the spectral dependence of that is typically approximated by a simple linear dependence , where and are empirical parameters. Actually, the exact value of is not needed in the derivation of (see Sec. 2 for details), whereas the value of is crucial. To overcome the assumption on , a broadband SRS approach can be implemented6,7 but that would require a more complex setup8 jeopardizing the compactness and simplicity of the SRS approach. Therefore, in most of the compact and wearable CW-NIRS devices, a minimum set of two wavelengths are implemented, making the assumption on rather critical. Moreover, during a prolonged measurement, is usually kept constant, whereas it has not yet been perfectly understood whether variations of the structural organization of the tissue itself can induce changes in .9,10 To our knowledge, a detailed study on the effect of on SRS still has not been conducted, whereas this problem has been tackled in the context of TD NIRS11,12 or FD NIRS.13 A second important assumption of the SRS method is that the probed medium is spatially homogeneous, that is, the optical properties do not vary with the source-detector distance and with the depth from the surface. On the contrary, tissue homogeneity in depth is frequently violated in skeletal muscle oximetry, because of the presence of the adipose tissue over the muscle, and in cerebral oximetry, due to the layered structure of the human head (with scalp, skull, cerebrospinal fluid, gray matter, and white matter). In CW-NIRS, to tackle tissue heterogeneity, a multi-distance or tomographic scheme should be implemented, again at the cost of increased complexity of the device.14 Adding a short source-detector distance channel () to sample superficial tissue hemodynamics is a solution recommended in functional NIRS setups.15 However, to our knowledge, this approach has not been implemented in SRS NIRS. “Few works have investigated the effect of superficial adipose tissue on SRS results16,17 and the effect of a layered medium with different optical properties,6,18 whereas a systematic study is still missing. An additional minor limitation of the SRS NIRS approach is the use of the so-called “zero-boundary” condition (ZBC) that proved to be less accurate for describing light propagation at tissue boundaries.19 This study aims to explore the accuracy and robustness of the SRS NIRS approach for estimating hemodynamic parameters, in particular the . We will first recall the basic theory behind SRS, then we will study the accuracy and the robustness of the SRS NIRS approach for the estimate of and of the hemodynamic parameters by means of numerical simulations in the framework of the photon diffusion theory. 2.TheoryThe SRS approach models the tissue as a semi-infinite medium with absorption coefficient and reduced scattering coefficient , and it adopts a reflectance geometry configuration with a collimated light source and a source detector distance . Under the extrapolated boundary condition (EBC), the CW reflectance (the number of photons per unit area exiting the medium) at a distance is given by where , , is the extrapolation distance, is the diffusion coefficient, is the effective attenuation coefficient, and is a parameter accounting for refractive index mismatch at the medium surface.20Both the original derivation of the SRS method4 and the recent developments7 employ the simpler ZBC obtained by setting . Despite the EBC solution for the reflectance is known to be more accurate than the ZBC solution, it was demonstrated that for the SRS approach, the use of the EBC yields no significant gain over the ZBC at the cost of an increased complexity in the computation.7 By adopting the ZBC and applying the common approximation , we have Light attenuation is defined as . The SRS method derives from , the spatial derivative of as follows: Note that often in the implementation of the SRS method, the additional approximation is commonly used, leading to a simpler formula for However, there is no significant reduction in complexity or computation time using Eq. (4) with respect to Eq. (3), therefore in the following we will always use Eq. (3). Combining Eq. (3) with the definition of , we can derive the absorption coefficient, typically expressed as a function of wavelength () and experiment time () The a priori knowledge of is needed to derive . In the classical SRS approach, a constant value for is used, typically approximated as4 If we are only interested in deriving , then we can simply assume and leave unknown. By the Beer’s law and knowing the specific absorption coefficients and ,21 Eqs. (5) and (6) yield , from which we can derive and . Therefore, the parameter simplifies in the derivation of . In the Biomedical Optics community, the spectral feature of the reduced scattering coefficient is typically approximated by means of an empirical power law derived from Mie’s theory as where is a reference wavelength, is related to the volume density of equivalent scatterers, and is the so-called scatter power coefficient that depends on the size of the equivalent scatterers.22The linear approximation for employed in the SRS approach can be interpreted as a first order approximation of Eq. (7). Using the classic Taylor expansion method for around a given , one obtains in fact 3.Material and Methods3.1.SimulationsWe simulated a hemodynamic experiment (total duration 39 min, with time points every 1 min) with changes in the concentration of and HHb mimicking realistic conditions for a skeletal muscle (e.g., deoxygenation following arterial cuff occlusion, hyperemic peak after cuff release, venous occlusion), as shown in Fig. 1. Baseline values () were , , , and . Fig. 1The nominal values of used in the simulations of Secs. 4.1–4.3 and 4.5. The shaded yellow areas represent the AO and VO tests. ![]() In a first set of simulations, from to , we mimicked an arterial occlusion (AO), with tHb kept constant at baseline value and progressively reducing to 8.3%, followed from to by the hyperemic reperfusion and return to baseline values. Then, from to , we mimicked a venous occlusion (VO), with kept constant at baseline value and a linear increase of tHb up to , followed by a reversed change mimicking a reduction of perfusion with constant followed by a return to baseline values. The absorption coefficients at 760 and 850 nm (see Fig. 2) were then derived as linear combination of the concentrations of and HHb by means of the Beer’s law with the knowledge of the specific absorption coefficients and .21 Fig. 2The nominal values of (a) and (b) used in the simulations of Secs. 4.1–4.3 and 4.5. Red 760 nm, purple 850 nm. The shaded yellow areas represent the AO and VO tests. ![]() The baseline reduced scattering coefficient was calculated by means of Eq. (7), assuming , , and , resulting in 1.0 and at 760 and 850 nm, respectively. Once the optical properties were obtained, we used analytical solutions of the photon diffusion equation in the EBC approximation in different geometries to calculate the CW reflectance at three source-detector distances (, 40, 45 mm) at wavelengths 760 and 850 nm.20,23 In the first set of simulations, a semi-infinite medium was considered, and the reduced scattering coefficient was kept constant at the baseline value (see Secs. 4.1–4.3). In a second simulation, a semi-infinite medium was considered, and the reduced scattering coefficient was linearly varied with respect to the initial value during the experiment time (see Sec. 4.4), whereas the hemodynamic parameters were maintained constant. Finally, a two-layer medium was considered23 (two-layer cylinder with different thicknesses of the upper layer: , 7.5, 10 mm and total thickness ), and hemodynamic changes were applied either to the upper or to the lower medium layer while keeping the hemodynamic parameters of the other layer constant at the baseline values (see Sec. 4.5). A schematic of the main parameters used in the forward simulations is reported in Table 1. Table 1Details on the main parameters used in the forward simulations and in the SRS approach.
NA, not applicable. 3.2.Data AnalysisFrom the simulated CW reflectance at different source detector distances , we derived the spatial derivative of the attenuation by means of central finite differences as , with and the shortest and longest source detector distances, respectively. As clearly described by,14 in the practical implementation when using two source detector distances and , with , the numerical approximation can be used in Eq. (3). Applying Eq. (5), it was then possible to derive the estimate of , provided the assumption on given by Eq. (7) or Eq. (8). Then, the hemodynamic parameters were derived by inverting the Beer’s law. A schematic of the parameters employed in the data analysis is also reported in Table 1. 4.Results4.1.Case 1: Semi-Infinite Medium and Exact Values for the Reduced Scattering CoefficientFor a preliminary test, we simulated CW reflectance data for a semi-infinite medium with EBC, and we estimated the hemodynamic parameters by means of the SRS approach assuming the exact knowledge of the reduced scattering coefficient [Eq. (5)]. In this way, the discrepancy between estimated and nominal values can be attributed to the use of the ZBC and to the simplifying approximation . In Fig. 3, the filled circle data points (•) report the results for the hemodynamic parameters. Figure 4 shows the corresponding relative error with respect to the nominal values. The estimates of the hemodynamic parameters are quite good, being the modulus of the relative error for lower than 1% for , whereas for lower , values it increases to about 8%. Errors for , HHb, and tHb stay within about 2%, 1% and 2%, respectively, for while reaching about 8%, 4% and 3% for lower values. 4.2.Case 2: Semi-Infinite Medium and Approximated Formula for the Reduced Scattering CoefficientThe same data of Sec. 4.1 were used assuming the linear approximation for the reduced scattering coefficient described in Eq. (8). We chose , average of 760 and 850 nm, yielding for the baseline value and . This approximation introduces a very small relative error (about ) on the reduced scattering coefficient at both wavelengths, therefore the performance of the SRS approach is not appreciably changed (data not shown). 4.3.Case 3: Semi-Infinite Medium and Over/Underestimation of the Reduced Scattering CoefficientThe same data of Sec. 4.1 were used assuming a wrong value for the reduced scattering coefficient . Considering Eqs. (7) and (8), the values for can be set in different ways. Assuming a wrong value for the parameter , it is equivalent in assuming a wrong value for parameter [see eq. (8)]. This affects both and HHb and consequently also tHb as shown in Figs. 3 and 4. A 20% overestimation (underestimation) error on parameter adds approximately an additional 20% overestimation (underestimation) on , HHb, and tHb. However, as expected, the error on has no influence on . Assuming a wrong value for parameter , it is equivalent to simultaneously changing parameter and parameter . A 20% underestimation (overestimation) in parameter yields approximately a 10% overestimation (underestimation) error in parameter and parameter . Overall, that has a smaller influence on all hemodynamic parameters including , , and tHb, whereas the error on HHb is negligible, as shown in Figs. 5 and 6. Fig. 5SRS results for semi-infinite homogeneous medium. Filled circle (•) exact , empty diamond (⋄) 20% overestimation of parameter b, cross (X) 20% underestimation of parameter b. The solid line in each panel is the nominal value. The shaded yellow areas represent the AO and VO tests. ![]() Fig. 6Relative error for the SRS results for semi-infinite homogeneous medium. Filled circle (•) exact , empty diamond (⋄) 20% overestimation of parameter b, cross (X) 20% underestimation of parameter b. The shaded yellow areas represent the AO and VO tests. ![]() In the framework of the SRS approach, the assumption on parameter is crucial. Assuming a wrong value for parameter only () has large effect on all hemodynamic (data not shown). The modulus of the errors for and tHb is larger than 20%. A smaller error (10%) is obtained for HHb. The error on is around 5% for but rapidly increases above 10% for . 4.4.Case 4: Semi-Infinite Medium and Temporal Changes of the Reduced Scattering CoefficientNow, we suppose that the reduced scattering coefficient is not constant, but it changes with the experiment time according to the relation , where is a constant baseline value and is the change with respect to the baseline. It is straightforward to derive that if in the SRS approach, we assume the fixed value , then a wrong value for the absorption coefficient is obtained: , with . If the percentage change is the same at all wavelengths, the effect is similar as introducing an error in parameter or , therefore we expect no changes in , whereas we can obviously have changes in , HHb, and tHb. Conversely, if , the estimate of will be affected since that is equivalent to introducing an error in parameter or . After simulating a scenario with constant hemodynamic parameters and variable reduced scattering coefficient , we have estimated the hemodynamic parameters by assuming a constant , as shown in Fig. 7. In particular, in the forward simulation, linear changes with respect to the baseline were applied keeping fixed parameter and varying parameter up to . Then, we changed parameter up to while keeping constant parameter . Note that after Eq. (7), in this last case , the reduced scattering coefficient at 760 nm is kept constant. Fig. 7The solid lines show the nominal values of and used in the simulations of Sec. 4.4. Red 760 nm, purple 850 nm. The shaded yellow areas represent the different changes applied to the scattering parameters. In the right panel, the points show the values used in the SRS estimation. ![]() As expected, changes in parameter yield significant errors in the estimates of , HHb, and tHb (see Figs. 8 and 9). However, as expected, it does not affect the estimate of . Conversely, when parameter changes, we get an error up to about in the estimate of . 4.5.Case 5: Effect of the Presence of a Superficial LayerThe assumption of homogeneous medium is expected to be violated in most biomedical applications, such as skeletal muscle oximetry (due to the presence of an adipose tissue layer overlying the muscle) or cerebral oximetry (due to the presence of scalp, skull, and cerebrospinal fluid). We simulated the presence of a superficial layer with different thicknesses (5, 7.5, and 10 mm). In the first case, hemodynamic changes have been applied only in the deeper layer (see Figs. 10 and 11). In the second case, we applied changes only to the upper layer (see Figs. 12 and 13). In both cases, the simulated reduced scattering coefficient was the same in the two layers, and it was maintained constant in the simulations. Fig. 10SRS results for a bilayer with thickness of the upper layer (•) 5 mm, (⋄) 7.5 mm, (X) 10 mm. The solid line is the nominal value for the lower layer, the dashed line is the nominal value for the upper layer. The shaded yellow areas represent the AO and VO tests. Changes are applied to the lower layer only. ![]() Fig. 11Relative error for the SRS results for a bilayer with thickness of the upper layer (•) 5 mm, (⋄) 7.5 mm, (X) 10 mm. The shaded yellow areas represent the AO and VO tests. Changes are applied to the lower layer only. ![]() Fig. 12SRS results for a bilayer with thickness of the upper layer (•) 5 mm, (⋄) 7.5 mm, (X) 10 mm. In each panel, the solid line is the nominal value for the lower layer, the dashed line is the nominal value for the upper layer. Changes are applied to the upper layer only. ![]() Fig. 13Relative error with respect to the lower layer for the SRS results for a bilayer with thickness of the upper layer (•) 5 mm, (⋄) 7.5 mm, (X) 10 mm. Changes are applied to the upper layer only. ![]() The presence of a superficial layer with constant hemodynamic parameters greatly affects the SRS estimates of the hemodynamic parameters in the lower layer. We observe an overestimation (underestimation) of all hemodynamic parameters when an increase (decrease) with respect to the baseline is present. For a thin (5 mm) upper layer, the error on can be lower than 10%, but it significantly increases well over 20% for larger thicknesses. Conversely, if we apply hemodynamic changes only to the superficial layer, while we are interested in the deeper layer, significant errors appear in and all other hemodynamic quantities as soon as the superficial layer thickness exceeds 5 mm. In both cases, the simulated data were analyzed using the exact reduced scattering coefficients, therefore the errors were only related to the presence of the superficial layer. 5.Discussion5.1.Robustness of the SRS ApproachUnder the hypothesis of a semi-infinite homogeneous medium and assuming to exactly know the reduced scattering coefficient, the SRS approach provides quite accurate estimates not only of but also of and HHb. The use of the ZBC and of the simplifying approximation is therefore largely acceptable (as also stated by Ref. 7). The linear approximation for the spectral dependence of the reduced scattering coefficient can be well tolerated, provided the estimate of parameter is robust. Unfortunately, reported values for parameter are diverse, ranging from 24 to .14 Indeed, as shown in Eq. (8), parameter depends on parameter , related to equivalent scatterer size in the tissue. Literature data on parameter are scarce and extremely variable (see Table 3 in the review paper25), therefore this choice is crucial for the accuracy of the SRS approach. The results presented in Sec. 4.3 highlight the need to determine the exact parameter and the strong error obtained when this parameter is approximated. Changes in the reduced scattering coefficients may affect the estimate of especially if unbalanced changes occur at the different wavelengths. If balanced, rather than unbalanced, changes will occur (e.g., if changes in scatterer density but not in scatter size occur), then the effect on the estimate of is negligible. Moreover, the results reported in Sec. 4.4 could be associated to inter-subject variability of scattering parameter (). Thus, the error reported in Figs. 3 and 5 (5% for , and more than 10% for ) could be representative of the mean error performed when multiple subjects are measured. The strongest limitation of the SRS approach is the assumption on the homogeneity of the medium. The presence of a superficial layer affects the estimates of independently from which layer the hemodynamic changes occur. A priori estimation of the thickness of the upper layer can be easily obtained with a plicometer or even better with ultrasonography. Correction factors for the presence of upper layer with different optical properties could be implemented either by adopting more accurate physical models for photon migration, such as solution of the photon diffusion equation in a two-layer medium,23 or applying empirical strategies based on phantom16 or in vivo measurements.26 An indicator of the quality and robustness of the SRS estimate for in case of tissue heterogeneity can be obtained adopting more than two source detector distances and comparing the absorption in the tissue over multiple distances.27 However, we stress that this is not a correction but simply an indicator of measurement quality. The presence of other tissue chromophores, such as melanin or bilirubin, can further reduce the accuracy of the CW-NIRS SRS method, if not properly considered, such as for pulse oximetry.28,29 These chromophores are typically found in the epidermis; therefore, despite the limited thickness of this layer, all detected photons will be affected. Possible solutions to circumvent the problem of skin pigmentation variance in individuals can be the use of more wavelengths to spectrally discriminate the chromophores30,31 and the use of more complex modeling (e.g., three-layer geometry).23 In this study, source detector distances and light wavelengths were chosen in accordance with CW-NIRS SRS devices. Indeed, clinical instruments for CW-NIRS SRS use sensors with different source detector distances tailored to the application: for neonatal monitoring a distance of 25 mm is used,32 for adult monitoring a distance of 40 or 50 mm is preferred,33,34 whereas intermediate values are sometimes employed for pediatric population. Increasing source detector distance aims at boosting the photon penetration depth,35 therefore improving sensitivity to the deeper tissues, such as brain cortex below scalp and skull or muscle below the adipose tissue. We highlight that in this work, we have not considered a specific device but simply the SRS method. For this reason, we have not introduced in the simulation any source of instrumental noise. Indeed, noise level in CW-NIRS devices is well tolerable unless extreme conditions (e.g., too high absorption) are considered. Specific simulations should be performed in that case. The performance of a real SRS device can be ameliorated by adopting specific calibration methods. Companies providing CW-NIRS devices exploit different calibration methods, which are rarely described. As an example, Ref. 36 reports a calibration for CW-NIRS devices with in vivo measurements. They consider the tissue , where is the arterial saturation and the jugular venous saturation. And they consider a linear factor between the measured and expected saturation: . Those factors, however, cannot be found in literature and make it very hard to reproduce real-life scenarios. Thus, in this work, we focused on the limitations due to the theoretical model, which appear to be not negligible. Finally, we observe that hemodynamic parameters in this work were tailored to the problem of monitoring skeletal muscle oxidative metabolism. Baseline values for geometrical, optical, and hemodynamic parameters are indeed realistic being reported by many authors (see, for example, Refs. 6, 7, 13). Conversely, the simulated 8 min duration of the vascular occlusions can be considered as an upper limit, useful to test extreme values of the optical and hemodynamic parameters. In case of cerebral oximetry, lower baseline values for and HHb should be used, especially for neonates. However, we do not expect large differences in the results since most of the errors arise from the violation of the homogeneity of tissue structure that is crucial also for cerebral oximetry. 5.2.Considerations on the Differential Pathlength FactorIt is worth noting that, in principle, assuming values for and would allow one to also get an absolute estimate of the differential pathlength factor (DPF).37 The estimate of the DPF is generally taken from the literature (see, for example, Ref. 38) while it could be derived by the SRS approach as well as also noticed by Ref. 39. When is known, assuming both and is in fact equivalent to assume , and correspondingly equivalent to assume the DPF. In fact, from the photon diffusion theory20 in the ZBC approximation and with , we have As expected, the estimate of the DPF is strongly affected by errors in parameter (or equivalently ) (see Fig. 14 top row), whereas it is less affected by errors in parameter (or ) (see Fig. 14 middle row). The presence of a superficial layer introduces significant errors in the estimate of DPF (see Fig. 14 bottom row). Fig. 14DPF estimate from Eq. (9). (a) Errors in parameter a (⋄) , (X) +20%; (b) errors in parameter b (⋄) , (X) +20%; (c) two-layer medium [thickness of upper layer (•) 5 mm, (⋄) 7.5 mm, (X) 10 mm] with changes in the lower layer and upper layer with constant optical and hemodynamic properties; and (d) two-layer medium [thickness of upper layer (•) 5 mm, (⋄) 7.5 mm, (X) 10 mm] with changes in the upper layer and lower layer with constant optical and hemodynamic properties. Solid lines are exact values. ![]() 5.3.Considerations on the Modified Beer Lambert LawAbsolute estimates of and HHb are generally not derived by SRS devices since and (i.e., changes with respect to an arbitrary baseline) are usually obtained by the modified Beer Lambert (MBL) law.38 In the MBL law, changes in the absorption coefficient with respect to an arbitrary fixed baseline are derived from changes in light attenuation when using a single source detector distance (the center distance in the SRS scheme) approach for CW-NIRS, provided the parameter the DPF is known40 where is the mean photon pathlength in the medium.From at (least) two wavelengths, one can finally get and . Indeed, assuming values for and would allow one to also get an absolute estimate of and HHb through Eq. (5). 6.ConclusionIn this work, we have recalled the theoretical basis of the CW SRS NIRS approach that is widely used in many commercial tissue oximeters, and we have studied by means of numerical simulations the robustness of the estimate of the hemodynamic parameters. We have shown the effect of errors in the assumption of the reduced scattering coefficient values and of the presence of tissue heterogeneity. We have also reported on the link between SRS parameters and and other important parameters in NIRS, such as the DPF, and the Mie parameters and . Users should be aware of the limitations intrinsic to the SRS approach. More sophisticated strategies could be considered for CW-NIRS, such as the use of self-calibrating method or the use of multiple short and long distances.14 DisclosuresDavide Contini and Alessandro Torricelli are co-founders of PIONIRS, spin-off company of the Politecnico di Milano. Code, Data, and Materials AvailabilityThe codes and datasets generated during and analyzed during the current study are available from the corresponding author upon reasonable request. AcknowledgmentsThis research was partially funded by the Horizon 2020 Framework Program of the European Union (Grant No. 863087), Horizon 2020 research and innovation program of the European Union (Grant No. 101016087), and MIUR-PRIN2020, “Neuromuscular impairment in aging: a longitudinal study of structural and functional mechanistic bases of age-related alterations (Trajector-AGE)” (Grant No. 2020477RW5PRIN). ReferencesJ. Ali et al.,
“Near-Infrared Spectroscopy (NIRS) for cerebral and tissue oximetry: analysis of evolving applications,”
J. Cardiothorac. Vasc. Anesth., 36
(8 Pt A), 2758
–2766 https://doi.org/10.1053/j.jvca.2021.07.015 JCVAEK 1053-0770
(2022).
Google Scholar
M. Ferrari, M. Muthalib and V. Quaresima,
“The use of near-infrared spectroscopy in understanding skeletal muscle physiology: recent developments,”
Phil. Trans. R. Soc. A, 369 4577
–4590 https://doi.org/10.1098/rsta.2011.0230 PTRMAD 1364-503X
(2011).
Google Scholar
D. Contini et al.,
“Brain and muscle near infrared spectroscopy/imaging techniques,”
J. Near Infrared Spectrosc., 20 15
–27 https://doi.org/10.1255/jnirs.977
(2012).
Google Scholar
S. Suzuki et al.,
“Tissue oxygenation monitor using NIR spatially resolved spectroscopy,”
Proc. SPIE, 3597 582
–592 https://doi.org/10.1117/12.356862
().
Google Scholar
K. Cowdrick,
“NIRS & DCS commercially available product landscape,”
(2023). https://datastudio.google.com/reporting/5f333396-7078-4f31-9056-e0f926b906f7/page/XcObC Google Scholar
J. D. Veesa and H. Dehghani,
“Functional near infrared spectroscopy using spatially resolved data to account for tissue scattering: a numerical study and arm-cuff experiment,”
J. Biophotonics, 12 e201900064 https://doi.org/10.1002/jbio.201900064
(2019).
Google Scholar
Z. Kovacsova et al.,
“Absolute quantification of cerebral tissue oxygen saturation with multidistance broadband NIRS in newborn brain,”
Biomed. Opt. Express, 12
(2), 907
–925 https://doi.org/10.1364/BOE.412088 BOEICL 2156-7085
(2021).
Google Scholar
G. Bale et al.,
“A new broadband near-infrared spectroscopy system for in-vivo measurements of cerebral cytochrome-c-oxidase changes in neonatal brain injury,”
Biomed. Opt. Express, 5
(10), 3450
–3466 https://doi.org/10.1364/BOE.5.003450 BOEICL 2156-7085
(2014).
Google Scholar
T. J. Barstow,
“Understanding near infrared spectroscopy (NIRS) and its application to skeletal muscle research,”
J. Appl. Physiol., 126
(5), 1360
–1376 https://doi.org/10.1152/japplphysiol.00166.2018
(2019).
Google Scholar
L. F. Ferreira, D. M. Hueber and T. J. Barstow,
“Effects of assuming constant optical scattering on measurements of muscle oxygenation by near-infrared spectroscopy during exercise,”
J. Appl. Physiol., 102
(1), 358
–367 https://doi.org/10.1152/japplphysiol.00920.2005
(2007).
Google Scholar
E. Ohmae et al.,
“Sensitivity correction for the influence of the fat layer on muscle oxygenation and estimation of fat thickness by time-resolved spectroscopy,”
J. Biomed. Opt., 19
(6), 067005 https://doi.org/10.1117/1.JBO.19.6.067005
(2014).
Google Scholar
I. Pirovano et al.,
“Effect of adipose tissue thickness and tissue optical properties on the differential pathlength factor estimation for NIRS studies on human skeletal muscle,”
Biomed. Opt. Express, 12
(1), 571
–587 https://doi.org/10.1364/BOE.412447 BOEICL 2156-7085
(2020).
Google Scholar
G. Blaney et al.,
“Broadband diffuse optical spectroscopy of two-layered scattering media containing oxyhemoglobin, deoxyhemoglobin, water, and lipids,”
J. Innov. Opt. Health Sci., 15
(3), 2250020 https://doi.org/10.1142/S1793545822500201
(2022).
Google Scholar
F. Scholkmann et al.,
“A review on continuous wave functional near-infrared spectroscopy and imaging instrumentation and methodology,”
Neuroimage, 85 6
–27 https://doi.org/10.1016/j.neuroimage.2013.05.004 NEIMEF 1053-8119
(2014).
Google Scholar
M. A. Yücel et al.,
“Best practices for fNIRS publications,”
Neurophotonics, 8
(1), 012101 https://doi.org/10.1117/1.NPh.8.1.012101
(2021).
Google Scholar
V. M. Niemeijer et al.,
“The influence of adipose tissue on spatially resolved near-infrared spectroscopy derived skeletal muscle oxygenation: the extent of the problem,”
Physiol. Meas., 38
(3), 539
–554 https://doi.org/10.1088/1361-6579/aa5dd5 PMEAE3 0967-3334
(2017).
Google Scholar
M. C. van Beekvelt et al.,
“Adipose tissue thickness affects in vivo quantitative near-IR spectroscopy in human skeletal muscle,”
Clin. Sci. (Lond.), 101
(1), 21
–28 https://doi.org/10.1042/cs1010021
(2001).
Google Scholar
D. Piao et al.,
“On the geometry dependence of differential pathlength factor for near-infrared spectroscopy. I. Steady-state with homogeneous medium,”
J. Biomed. Opt., 20
(10), 105005 https://doi.org/10.1117/1.JBO.20.10.105005 JBOPFO 1083-3668
(2015).
Google Scholar
R. C. Haskell et al.,
“Boundary conditions for the diffusion equation in radiative transfer,”
J. Opt. Soc. Am. A Opt. Image Sci. Vision, 11
(10), 2727
–2741 https://doi.org/10.1364/josaa.11.002727
(1994).
Google Scholar
D. Contini, F. Martelli and G. Zaccanti,
“Photon migration through a turbid slab described by a model based on diffusion approximation. I. Theory,”
Appl. Opt., 36 4587
–4599 https://doi.org/10.1364/AO.36.004587 APOPAI 0003-6935
(1997).
Google Scholar
S. Prahl,
“Optical absorption of hemoglobin,”
(2023). https://omlc.org/spectra/hemoglobin/ Google Scholar
J. R. Mourant et al.,
“Predictions and measurements of scattering and absorption over broad wavelength ranges in tissue phantoms,”
Appl Opt., 36
(4), 949
–957 https://doi.org/10.1364/AO.36.000949
(1997).
Google Scholar
A. Liemert and A. Kienle,
“Light diffusion in a turbid cylinder. II. Layered case,”
Opt. Express, 18
(9), 9266
–9279 https://doi.org/10.1364/OE.18.009266 OPEXFF 1094-4087
(2010).
Google Scholar
S. J. Matcher, M. Cope and D. T. Delpy,
“In vivo measurements of the wavelength dependence of tissue-scattering coefficients between 760 and 900 nm measured with time-resolved spectroscopy,”
Appl. Opt., 36
(1), 386
–396 https://doi.org/10.1364/AO.36.000386
(1997).
Google Scholar
S. L. Jacques,
“Optical properties of biological tissues: a review,”
Phys. Med. Biol., 58
(11), R37
–R61 https://doi.org/10.1088/0031-9155/58/11/R37 PHMBA7 0031-9155
(2013).
Google Scholar
P. E. Bickler, J. R. Feiner and M. D. Rollins,
“Factors affecting the performance of 5 cerebral oximeters during hypoxia in healthy volunteers,”
Anesth. Analg., 117
(4), 813
–823 https://doi.org/10.1213/ANE.0b013e318297d763
(2013).
Google Scholar
X. Sun et al.,
“Skin pigmentation interferes with the clinical measurement of regional cerebral oxygen saturation,”
Br. J. Anaesth., 114
(2), 276
–280 https://doi.org/10.1093/bja/aeu335 BJANAD 0007-0912
(2015).
Google Scholar
P. Silverston, M. Ferrari and V. Quaresima,
“Pulse oximetry and the pandemic,”
BMJ, 378 e071474 https://doi.org/10.1136/bmj-2022-071474
(2022).
Google Scholar
A. Ferraris, M. Jacquet-Lagrèze and J. L. Fellahi,
“Four-wavelength near-infrared peripheral oximetry in cardiac surgery patients: a comparison between EQUANOX and O3,”
J. Clin. Monit. Comput., 32
(2), 253
–259 https://doi.org/10.1007/s10877-017-0025-z
(2018).
Google Scholar
, “ForeSight tissue oximetry system: understanding the role of melanin in tissue oximetry, NP--EU-0745 v1.0,”
https://education.edwards.com/foresight-elite-white-paper-melanin-and-tissue-oximetry/953858#
(2021).
Google Scholar
M. L. Hansen et al.,
“Cerebral oximetry monitoring in extremely preterm infants,”
N. Engl. J. Med., 388
(16), 1501
–1511 https://doi.org/10.1056/NEJMoa2207554 NEJMAG 0028-4793
(2023).
Google Scholar
H. Ayaz et al.,
“Optical imaging and spectroscopy for the study of the human brain: status report,”
Neurophotonics, 9
(Suppl. 2), S24001 https://doi.org/10.1117/1.NPh.9.S2.S24001
(2022).
Google Scholar
B. Grassi and V. Quaresima,
“Near-infrared spectroscopy and skeletal muscle oxidative function in vivo in health and disease: a review from an exercise physiology perspective,”
J. Biomed. Opt., 21 091313 https://doi.org/10.1117/1.JBO.21.9.091313 JBOPFO 1083-3668
(2016).
Google Scholar
F. Martelli et al.,
“There’s plenty of light at the bottom: statistics of photon penetration depth in random media,”
Sci. Rep., 6 27057 https://doi.org/10.1038/srep27057 SRCEC3 2045-2322
(2016).
Google Scholar
V. Pollard et al.,
“Validation in volunteers of a near-infrared spectroscope for monitoring brain oxygenation in vivo,”
Anest. Analg., 82
(2), 269
–277 https://doi.org/10.1097/00000539-199602000-00010
(1996).
Google Scholar
A. Duncan et al.,
“Optical pathlength measurements on adult head, calf and forearm and the head of the newborn infant using phase resolved optical spectroscopy,”
Phys. Med. Biol., 40
(2), 295
–304 https://doi.org/10.1088/0031-9155/40/2/007 PHMBA7 0031-9155
(1995).
Google Scholar
F. Scholkmann and M. Wolf,
“General equation for the differential pathlength factor of the frontal human head depending on wavelength and age,”
J. Biomed. Opt., 18
(10), 105004 https://doi.org/10.1117/1.JBO.18.10.105004 JBOPFO 1083-3668
(2013).
Google Scholar
A. M. Chiarelli et al.,
“Differential pathlength factor in continuous wave functional near-infrared spectroscopy: reducing hemoglobin’s cross talk in high-density recordings,”
Neurophotonics, 6
(3), 035005 https://doi.org/10.1117/1.NPh.6.3.035005
(2019).
Google Scholar
F. Scholkmann, A. J. Metz and M. Wolf,
“Measuring tissue hemodynamics and oxygenation by continuous-wave functional near-infrared spectroscopy - how robust are the different calculation methods against movement artifacts,”
Physiol. Meas., 35
(4), 717
–734 https://doi.org/10.1088/0967-3334/35/4/717 PMEAE3 0967-3334
(2014).
Google Scholar
BiographyCaterina Amendola is a dedicated postdoctoral researcher specializing in the field of diffuse optics. She works in the Physics Department of Politecnico di Milano (Italy), where she earned her PhD in March 2022. Her research primarily focuses on time-domain near-infrared spectroscopy (TD-NIRS) and diffuse correlation spectroscopy (DCS) techniques. With expertise in developing hybrid TD-NIRS and DCS devices, she applies these advancements in clinical settings while conducting data analysis and physical modelling. Davide Contini received his MS degree in electronic engineering and his PhD in physics from Politecnico di Milano, Italy, in 2004 and 2007, respectively. He is an associate professor in the Department of Physics, Politecnico di Milano. He has authored more than 150 papers in international peer-reviewed journals and conference proceedings. His research activity is focused on time-resolved spectroscopy of highly diffusive media for applications in biology and medicine. Rebecca Re is an associate professor at the Physics Department of Politecnico di Milano (Italy). Her research activity focuses on light propagation in turbid media with particular interest in biological tissues. In particular, she employs time domain near infrared spectroscopy (TD NIRS) and diffuse correlation spectroscopy (DCS) to investigate brain hemodynamics and muscle oxidative metabolism and microcirculation in healthy subjects and patients. Her expertise ranges from the devices development to data analysis and physical models. Lorenzo Spinelli received his MS and PhD degrees in physics from the University of Milan, Italy, in 1994 and 1999, respectively. He devoted his activity research to the study of structures developing in the section of broad area radiation beams. In 2001, he became a researcher for the Italian Research National Council at the Politecnico of Milan. His current research interest is the study of photon migration in turbid media for optical biopsy and imaging. Lorenzo Frabasile received his master’s degree in engineering physics from Politecnico di Milano, Italy, in 2020. He is pursuing a PhD in engineering physics at Politecnico di Milano. His research is focused on the optical characterization of biological tissues by time-domain near infrared spectroscopy. Pietro Levoni received his BS and MS degrees in engineering physics from Politecnico di Milano, Italy, in 2017 and 2020, respectively. He is currently pursuing a PhD in physics at Politecnico di Milano. His research focuses on optical characterization of turbid media by time-domain near infrared spectroscopy. Alessandro Torricelli is a full-time professor in the Department of Physics, Politecnico di Milano, Italy. He received his MS degree in electronic engineering from Politecnico di Milano in 1994, and his PhD in physics from Politecnico di Torino, Italy, in 1999. He is the author of more than 200 papers in international peer-reviewed journals. His current research interests include photon migration in diffusive media, functional near-infrared spectroscopy, and noninvasive diffuse spectroscopy with time-domain systems. |
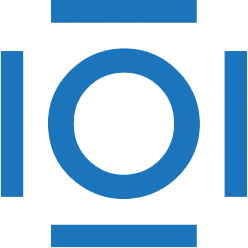
CITATIONS
Cited by 4 scholarly publications.
Tissues
Hemodynamics
Scattering
Near infrared spectroscopy
Oxygenation
Error analysis
Simulations