|
ACCESS THE FULL ARTICLE
No SPIE Account? Create one
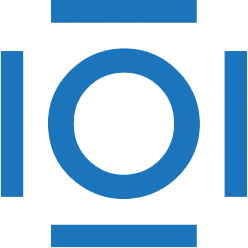
CITATIONS
Cited by 1 scholarly publication.
Machine learning
Sensors
Artificial neural networks
Structural health monitoring
Signal processing
Visualization
Nondestructive evaluation