|
ACCESS THE FULL ARTICLE
No SPIE Account? Create one
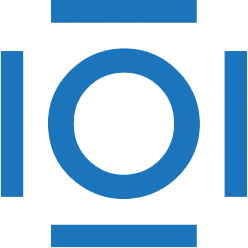
CITATIONS
Cited by 7 scholarly publications and 1 patent.
Image segmentation
Convolutional neural networks
Computer programming
Image classification
Classification systems
Computer vision technology
Convolution