|
ACCESS THE FULL ARTICLE
No SPIE Account? Create one
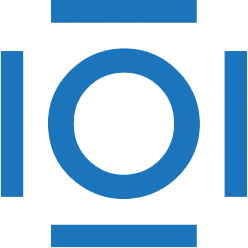
CITATIONS
Cited by 1 scholarly publication and 2 patents.
Image segmentation
X-rays
X-ray imaging
3D acquisition
Arteries
Computer programming
Image processing algorithms and systems