|
ACCESS THE FULL ARTICLE
No SPIE Account? Create one
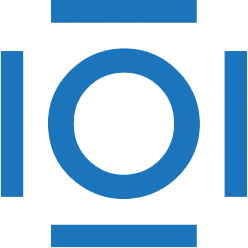
CITATIONS
Cited by 2 scholarly publications.
Tumors
Image quality
Motion models
Computed tomography
CT reconstruction
Medical imaging
Lung cancer