|
ACCESS THE FULL ARTICLE
No SPIE Account? Create one
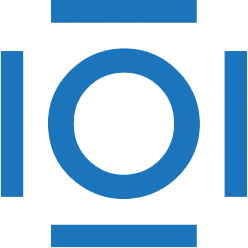
CITATIONS
Cited by 4 scholarly publications.
Convolution
Neural networks
Convolutional neural networks
Artificial neural networks
Image processing
Optimization (mathematics)
Machine learning