|
ACCESS THE FULL ARTICLE
No SPIE Account? Create one
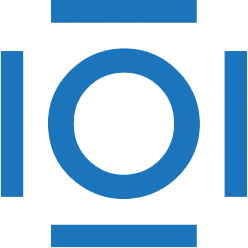
CITATIONS
Cited by 1 scholarly publication.
Convolution
Feature extraction
Annealing
Evolutionary algorithms
Neural networks