|
ACCESS THE FULL ARTICLE
No SPIE Account? Create one
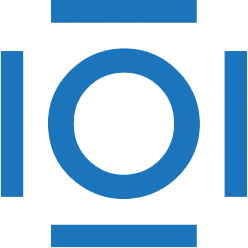
CITATIONS
Cited by 5 scholarly publications.
Neurons
Denoising
Computed tomography
Convolution
Surgery
Computer programming
Feature extraction