|
ACCESS THE FULL ARTICLE
No SPIE Account? Create one
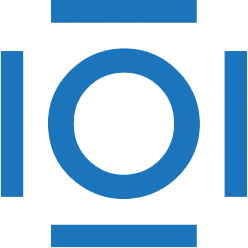
CITATIONS
Cited by 1 scholarly publication.
Data modeling
Image classification
Network architectures
Image processing
Machine learning
Performance modeling
Data conversion