|
ACCESS THE FULL ARTICLE
No SPIE Account? Create one
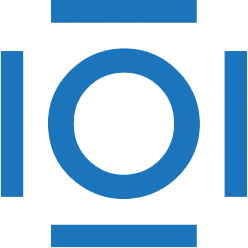
CITATIONS
Cited by 1 scholarly publication.
Synthetic aperture radar
Radon transform
Image restoration
Computed tomography
X-ray computed tomography
Data modeling
Image quality