|
ACCESS THE FULL ARTICLE
No SPIE Account? Create one
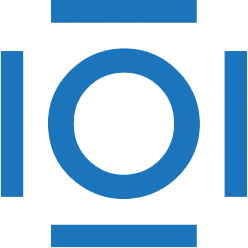
CITATIONS
Cited by 1 scholarly publication.
Image segmentation
Tissues
Breast
Magnetic resonance imaging
Data modeling
Image enhancement
Visualization