|
ACCESS THE FULL ARTICLE
No SPIE Account? Create one
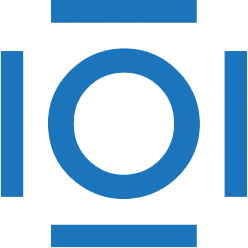
CITATIONS
Cited by 4 scholarly publications.
Radar
Neural networks
Extremely high frequency
Automatic target recognition
Signal processing
Systems modeling
Sensors