|
ACCESS THE FULL ARTICLE
No SPIE Account? Create one
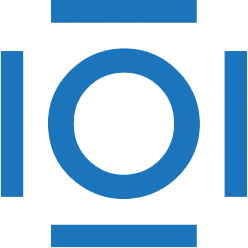
CITATIONS
Cited by 5 scholarly publications.
Instrument modeling
Statistical modeling
Process control
Control systems
Photoresist materials
Data modeling
Semiconducting wafers