|
ACCESS THE FULL ARTICLE
No SPIE Account? Create one
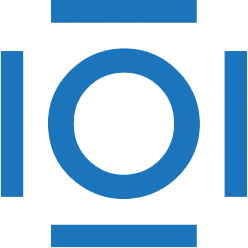
CITATIONS
Cited by 2 scholarly publications.
Data modeling
Tumor growth modeling
Breast
Cancer
Correlation function
Diagnostics
Statistical modeling