|
ACCESS THE FULL ARTICLE
No SPIE Account? Create one
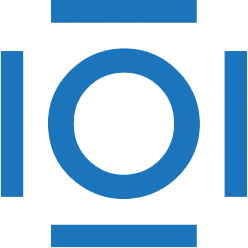
CITATIONS
Cited by 1 scholarly publication.
Data modeling
Expectation maximization algorithms
Optimization (mathematics)
Stochastic processes
Statistical analysis
Statistical modeling
Systems modeling