|
ACCESS THE FULL ARTICLE
No SPIE Account? Create one
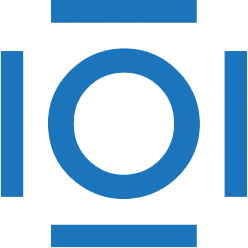
CITATIONS
Cited by 13 scholarly publications.
Detection and tracking algorithms
Visualization
Optical tracking
Calibration
Sensors
Image processing
Principal component analysis