|
ACCESS THE FULL ARTICLE
No SPIE Account? Create one
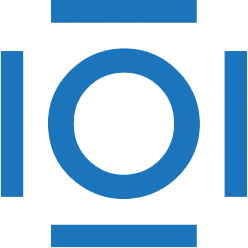
CITATIONS
Cited by 6 scholarly publications.
Detection and tracking algorithms
Target detection
Neural networks
Target recognition
Image classification
Image processing
Algorithm development