|
ACCESS THE FULL ARTICLE
No SPIE Account? Create one
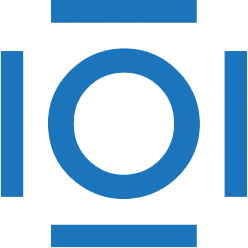
CITATIONS
Cited by 7 scholarly publications.
Statistical modeling
Data modeling
Systems modeling
Complex systems
Prototyping
Neural networks
Statistical analysis