|
ACCESS THE FULL ARTICLE
No SPIE Account? Create one
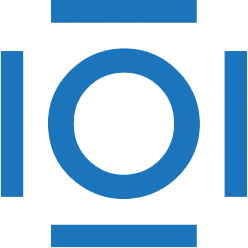
CITATIONS
Cited by 4 scholarly publications.
Logic
Error analysis
Associative arrays
Data communications
Data modeling
Feature extraction
Matrices