|
ACCESS THE FULL ARTICLE
No SPIE Account? Create one
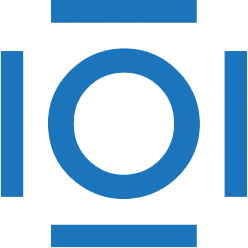
CITATIONS
Cited by 12 scholarly publications.
Data modeling
Spatial frequencies
Mammography
Visualization
Composites
Diagnostics
Signal detection