|
ACCESS THE FULL ARTICLE
No SPIE Account? Create one
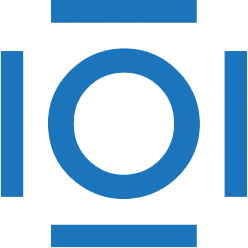
CITATIONS
Cited by 11 scholarly publications.
Aerosols
Atmospheric corrections
Water
Ocean optics
Atmospheric modeling
Neural networks
Evolutionary algorithms