|
ACCESS THE FULL ARTICLE
No SPIE Account? Create one
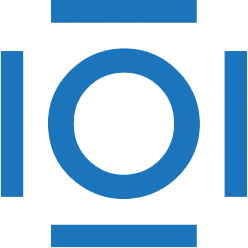
CITATIONS
Cited by 8 scholarly publications.
Data modeling
Denoising
Statistical modeling
Stochastic processes
Performance modeling
Image segmentation
Image processing