|
ACCESS THE FULL ARTICLE
No SPIE Account? Create one
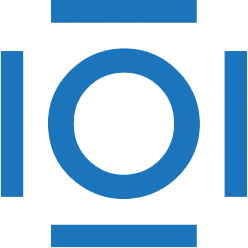
CITATIONS
Cited by 5 scholarly publications.
Control systems
Semiconducting wafers
Optical alignment
Overlay metrology
Error analysis
Manufacturing
Data modeling