|
ACCESS THE FULL ARTICLE
No SPIE Account? Create one
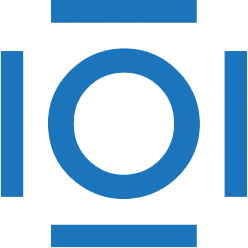
CITATIONS
Cited by 2 scholarly publications.
Neural networks
Systems modeling
Complex systems
System identification
Damage detection
Dynamical systems
Mechanics