|
ACCESS THE FULL ARTICLE
No SPIE Account? Create one
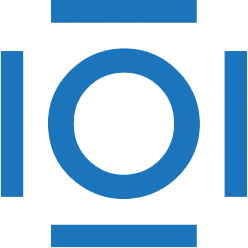
CITATIONS
Cited by 3 scholarly publications.
Performance modeling
Monte Carlo methods
Diagnostics
Diffractive optical elements
Algorithm development
Corrosion
Feature extraction