|
ACCESS THE FULL ARTICLE
No SPIE Account? Create one
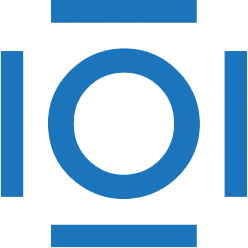
CITATIONS
Cited by 4 scholarly publications.
Wavelets
Error analysis
Data modeling
Functional magnetic resonance imaging
Neuroimaging
Wavelet transforms
Image analysis