|
ACCESS THE FULL ARTICLE
No SPIE Account? Create one
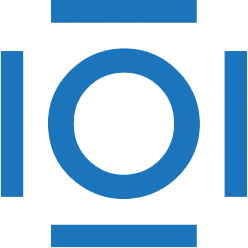
CITATIONS
Cited by 4 scholarly publications.
Fourier transforms
Binary data
Neodymium
Visualization
Computer simulations
Defect detection
Distributed computing