|
ACCESS THE FULL ARTICLE
No SPIE Account? Create one
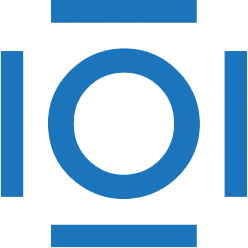
CITATIONS
Cited by 2 scholarly publications.
Organisms
Statistical modeling
Systems modeling
Cancer
Computer simulations
Control systems
Feedback signals