|
ACCESS THE FULL ARTICLE
No SPIE Account? Create one
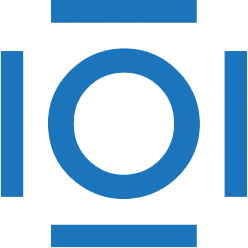
CITATIONS
Cited by 2 scholarly publications.
Vegetation
Binary data
Sensors
Statistical analysis
Image classification
Point spread functions
Data acquisition