|
ACCESS THE FULL ARTICLE
No SPIE Account? Create one
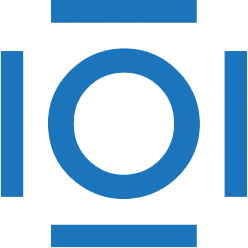
CITATIONS
Cited by 4 scholarly publications.
Autoregressive models
Principal component analysis
Data modeling
Acoustics
Signal detection
Pattern recognition
Roads