|
ACCESS THE FULL ARTICLE
No SPIE Account? Create one
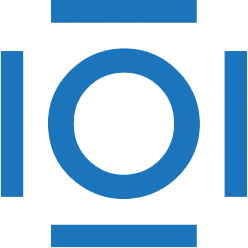
CITATIONS
Cited by 38 scholarly publications.
Signal to noise ratio
Radar
Data modeling
Imaging systems
Reconstruction algorithms
Data processing
Error analysis