|
ACCESS THE FULL ARTICLE
No SPIE Account? Create one
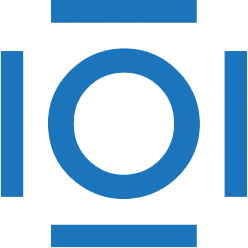
CITATIONS
Cited by 5 scholarly publications.
Target detection
Signal to noise ratio
Detection and tracking algorithms
Data conversion
Single mode fibers
Hyperspectral imaging
Image segmentation