|
ACCESS THE FULL ARTICLE
No SPIE Account? Create one
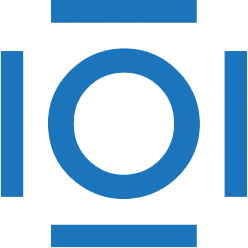
CITATIONS
Cited by 4 scholarly publications.
Reconstruction algorithms
X-ray computed tomography
X-rays
Diagnostics
Image restoration
X-ray imaging
Image filtering