|
ACCESS THE FULL ARTICLE
No SPIE Account? Create one
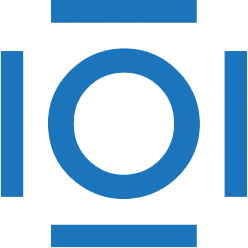
CITATIONS
Cited by 2 scholarly publications.
Brain
Neuroimaging
Matrices
Medicine
Positron emission tomography
Statistical analysis
Brain imaging