|
ACCESS THE FULL ARTICLE
No SPIE Account? Create one
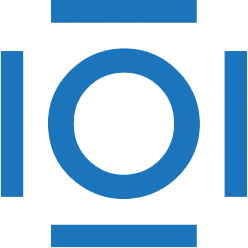
CITATIONS
Cited by 14 scholarly publications.
Thermal modeling
Principal component analysis
Data modeling
Signal to noise ratio
Nondestructive evaluation
Thermography
Aluminum