|
ACCESS THE FULL ARTICLE
No SPIE Account? Create one
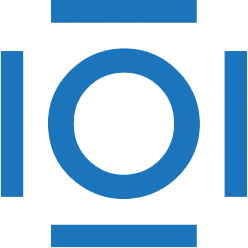
CITATIONS
Cited by 1 scholarly publication.
Data mining
Earth observing sensors
High resolution satellite images
Remote sensing
Visualization
Accuracy assessment
Algorithm development