|
ACCESS THE FULL ARTICLE
No SPIE Account? Create one
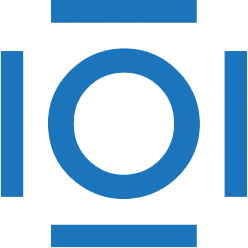
CITATIONS
Cited by 1 scholarly publication.
Medical imaging
Visualization
Image classification
Image segmentation
Image retrieval
Biomedical optics
Feature extraction