|
ACCESS THE FULL ARTICLE
No SPIE Account? Create one
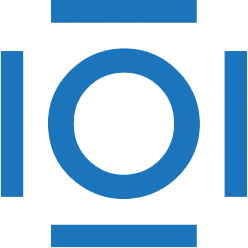
CITATIONS
Cited by 25 scholarly publications and 3 patents.
Image segmentation
Magnetic resonance imaging
Spine
3D modeling
Statistical analysis
Computed tomography
Feature extraction