|
ACCESS THE FULL ARTICLE
No SPIE Account? Create one
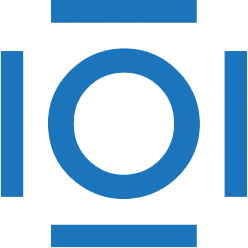
CITATIONS
Cited by 6 scholarly publications.
Sensors
Automatic target recognition
Data modeling
Feature extraction
Neural networks
Target detection
Detection and tracking algorithms