|
ACCESS THE FULL ARTICLE
No SPIE Account? Create one
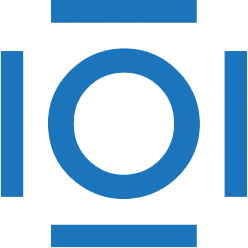
CITATIONS
Cited by 6 scholarly publications.
Electroluminescent displays
Tissues
Image analysis
Computing systems
Deconvolution
Scanners
Signal processing