|
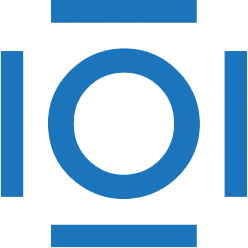
CITATIONS
Cited by 2 scholarly publications.
Polarimetry
Image classification
Principal component analysis
Classification systems
Synthetic aperture radar
Scattering
Feature extraction