|
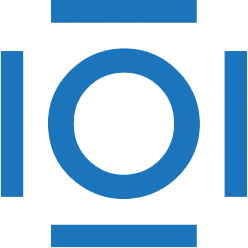
CITATIONS
Cited by 116 scholarly publications.
Vegetation
Reflectivity
Multispectral imaging
Data modeling
Agriculture
Machine learning
Short wave infrared radiation