Semantic segmentation is one of the most popular and challenging applications of deep learning. It refers to the process of dividing a digital image into semantically homogeneous areas with similar properties. We employ the use of deep learning techniques to perform semantic segmentation on high-resolution satellite images representing urban scenes to identify roads, vegetation, and buildings. A SegNet-based neural network with an encoder–decoder architecture is employed. Despite the small size of the dataset, the results are promising. We show that the network is able to accurately distinguish between these groups for different test images, when using a network with four convolutional layers. |
ACCESS THE FULL ARTICLE
No SPIE Account? Create one
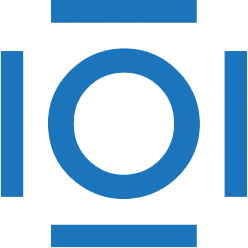
CITATIONS
Cited by 14 scholarly publications.
Image segmentation
Earth observing sensors
Satellite imaging
Satellites
Roads
Buildings
Vegetation