Reducing emissions from deforestation and forest degradation (REDD+) has emerged as a global climate change mitigation initiative under negotiation by the United Nations Framework Convention on Climate Change aimed at providing financial support to the developing countries for conserving respective forests. To implement the REDD+ initiative, developing countries need to estimate, among other necessitates, the activity data (i.e., pattern and process) of respective deforestation and forest degradation. Bangladesh is steadily progressing through its REDD+ roadmap. However, an important research issue to address includes using remote sensing technology to detect activity data for deforestation in a spatially explicit manner following the recommended good practice guidelines by the Intergovernmental Panel on Climate Change. This study mapped the activity data for deforestation of a mixed forest in Bangladesh during 1995 to 2015, applying Monte-Carlo spectral unmixing classification algorithm to Landsat images in CLASlite software. The classification was verified using independently drawn reference points from high-resolution Google Earth images. A postclassification comparison method was applied to generate landcover transition matrices. The outputs were highly accurate maps (overall accuracy >90 % ) and statics of activity data for deforestation of the study area. The approaches and findings may have significant implications in adopting any REDD+ project in Bangladesh. |
ACCESS THE FULL ARTICLE
No SPIE Account? Create one
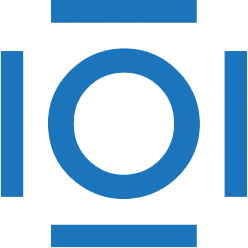
CITATIONS
Cited by 5 scholarly publications.
Earth observing sensors
Landsat
Error analysis
Data analysis
Image analysis
Clouds
Image classification